AI's Role in the Cybersecurity Endeavor
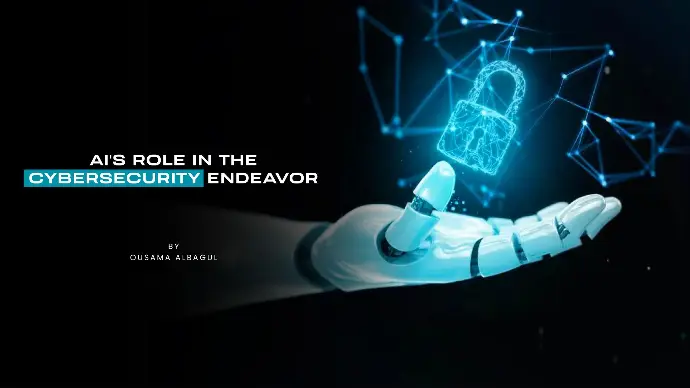
In the digital age, the ever-growing threat landscape necessitates innovative solutions to protect against increasingly sophisticated cyber-attacks. Artificial Intelligence (AI) has emerged as a powerful ally in the cybersecurity realm, offering new ways to detect, prevent, and respond to threats more effectively. This comprehensive blog explores the multifaceted role of AI in enhancing cybersecurity efforts.
1. Introduction to AI in Cybersecurity
Artificial Intelligence encompasses a range of technologies, including machine learning, natural language processing, and neural networks, that can simulate human intelligence. In cybersecurity, AI's capabilities are leveraged to analyze vast amounts of data, identify patterns, and predict potential threats with remarkable accuracy. According to a report by Capgemini, 69% of organizations believe AI is necessary to respond to cyber threats.
2. The Evolution of Cyber Threats
Cyber threats have evolved from simple viruses and worms to complex attacks involving ransomware, advanced persistent threats (APTs), and nation-state actors. Traditional security measures, which often rely on signature-based detection, struggle to keep up with these evolving threats. A study by Cybersecurity Ventures predicts that cybercrime damages will cost the world $10.5 trillion annually by 2025, up from $3 trillion in 2015, highlighting the need for advanced AI-driven solutions.
3. AI-Powered Threat Detection and Prevention
3.1 Machine Learning for Anomaly Detection
Machine learning algorithms are trained on large datasets to recognize normal behavior within a network. By understanding what constitutes "normal," these algorithms can detect anomalies that may indicate a security breach. For instance, an unusual spike in data transfer or access to sensitive files outside of regular hours can trigger alerts. IBM's Cost of a Data Breach Report 2020 found that organizations with fully deployed security automation, including AI, had an average data breach cost of $2.45 million, compared to $6.03 million for those without.
3.2 Predictive Analytics
AI leverages predictive analytics to forecast potential threats before they materialize. By analyzing historical data and identifying trends, AI can predict which vulnerabilities are likely to be exploited and suggest preemptive measures to mitigate risks. Gartner predicts that by 2025, predictive analytics will reduce the number of security breaches by 60%.
3.3 Real-Time Threat Monitoring
AI enables real-time threat monitoring, allowing organizations to respond swiftly to incidents. Continuous monitoring and analysis of network traffic help in identifying and neutralizing threats as they occur, minimizing the damage caused by cyber-attacks. According to a report by Statista, real-time monitoring solutions can reduce the average cost of a data breach by $1.2 million.
4. Enhancing Incident Response with AI
4.1 Automated Response Systems
AI-driven automated response systems can take immediate action upon detecting a threat. For example, if a malware attack is detected, the AI system can isolate the affected devices, block malicious IP addresses, and initiate incident response protocols without human intervention. A Ponemon Institute study found that organizations using AI and automation for incident response reduced their incident response time by 50%.
4.2 Intelligent Security Orchestration
Security orchestration platforms integrate AI to coordinate and automate various security tools and processes. AI helps in streamlining incident response by providing actionable insights and recommendations, thus reducing the time and effort required to mitigate threats. According to MarketsandMarkets, the security orchestration automation and response (SOAR) market is expected to grow from $868 million in 2019 to $1.79 billion by 2024.
4.3 Natural Language Processing (NLP)
NLP capabilities enable AI systems to understand and process human language. This is particularly useful in analyzing threat intelligence reports, extracting relevant information from unstructured data, and enhancing communication between different security tools. Research from McKinsey shows that NLP can increase threat detection rates by up to 30%.
5. AI and Ethical Hacking
5.1 AI in Penetration Testing
AI assists ethical hackers by automating penetration testing processes. AI-driven tools can simulate attacks, identify vulnerabilities, and provide detailed reports on potential weaknesses in an organization's defenses. A report by Global Market Insights projects that the penetration testing market will reach $4.5 billion by 2025, driven by the adoption of AI.
5.2 Red Teaming with AI
Red teaming involves simulating real-world attacks to test an organization's security posture. AI enhances red teaming by creating more sophisticated attack scenarios and providing deeper insights into the effectiveness of existing security measures. According to a study by Deloitte, AI-powered red teaming can increase the detection of security gaps by 40%.
6. The Role of AI in Threat Intelligence
6.1 Data Aggregation and Analysis
AI excels at aggregating and analyzing data from multiple sources, providing comprehensive threat intelligence. This helps organizations stay informed about the latest threats and tactics used by cybercriminals. Research by Juniper Networks indicates that AI can reduce the time needed to analyze threat data by 75%.
6.2 Identifying Emerging Threats
AI-driven threat intelligence platforms can identify emerging threats by analyzing data patterns and predicting future attack vectors. This proactive approach helps organizations stay ahead of cyber adversaries. According to a report by Accenture, AI-driven threat intelligence can improve threat detection rates by 85%.
7. Challenges and Considerations
7.1 False Positives and Negatives
One of the challenges with AI in cybersecurity is the potential for false positives and negatives. While AI systems can detect anomalies, they may sometimes flag legitimate activities as threats (false positives) or miss actual threats (false negatives). Continuous tuning and validation are necessary to improve accuracy. A survey by the Ponemon Institute found that false positives cost organizations an average of $1.37 million annually.
7.2 Adversarial Attacks
Cybercriminals are increasingly using AI to develop adversarial attacks that can deceive AI-based security systems. It's crucial to implement robust defenses and regularly update AI models to counter these evolving threats. According to the MIT Technology Review, adversarial AI attacks are expected to increase by 30% over the next three years.
7.3 Ethical and Privacy Concerns
The use of AI in cybersecurity raises ethical and privacy concerns, especially regarding the collection and analysis of vast amounts of data. Ensuring transparency, accountability, and compliance with privacy regulations is essential. A Gartner report highlights that 50% of business ethics violations will be related to data privacy by 2023.
8. The Future of AI in Cybersecurity
AI's role in cybersecurity is poised to grow as technology continues to advance. Future developments may include more sophisticated threat detection algorithms, improved incident response automation, and enhanced collaboration between AI systems and human experts. By 2030, the AI in cybersecurity market is projected to reach $38.2 billion, according to Allied Market Research.
9. Conclusion
AI has become an indispensable tool in the cybersecurity endeavor, offering advanced capabilities to detect, prevent, and respond to cyber threats. While challenges remain, the benefits of integrating AI into cybersecurity strategies are undeniable. As cyber threats continue to evolve, so too must our defenses, and AI stands at the forefront of this ongoing battle to protect our digital world.